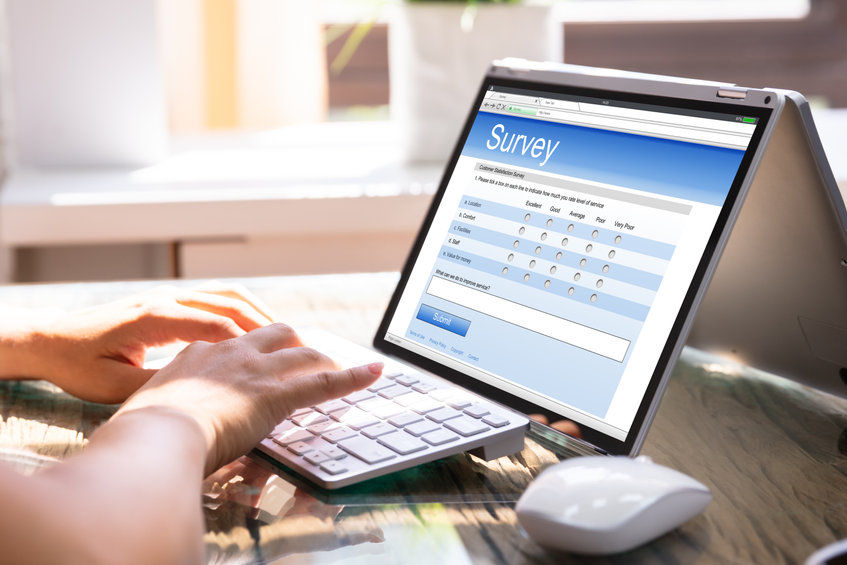
As experts in people, teams, and evaluating talent, we know there is no such thing as perfection. The best candidates are not the ones that are the most perfect in every way, they are the ones that best complement, augment, and resonate with or organizations and teams – bringing strengths that we need, while any weaknesses and gaps are covered by the strengths of others.
It is the same with data.
In the world of data collection, such as TA process feedback, there are two basic types of data that work together to form the perfect team – structured and unstructured. Both have value, both have strengths, and both have limitations. And like with any team, the key is to optimize to the strengths of each member while minimizing the impact of the weaknesses.
How do you design your feedback questions to best use both these data types?
The same way you do with any team. It starts with understanding each member, their strengths and limitations. Then identify how they can be best used together to reach a goal.
What is structured vs. unstructured data?
Structured data is a defined, limited set of information that can be easily organized, applied, and counted. Employer name, single- and multiple-choice selections, numbers, and email addresses are all examples of structured data.
Because the data is defined and limited, it’s easy to analyze.
For example.
Consider the question “which of the following choices is your favorite fruit – a) apples b) bananas c) oranges d) grapes.”
Even with thousands of answers, a few quick functions in a spreadsheet can easily count the responses for each by breaking it down to percentages of people who like apples, vs bananas, etc.
Add a little more structured data, such as the gender of the respondent, and I can compare the preference for apples based on gender.
But, what if the responder does not like any of those options, or doesn’t like fruit, or never tasted a banana so they have no idea what they taste like to compare them to apples? There is no option for any of that.
This is where unstructured data comes in.
Unstructured data is an undefined set of information. It has endless possibilities to capture every possible answer but requires interpretation to analyze. Open-ended questions are forms of unstructured data.
For example:
Consider the question “What is your favorite fruit?”
Responders can answer in any way they like. “I like both bananas and apples the same”, “I don’t like fruit”, “Kiwi”, “gummy bears”. The possibilities are limitless and the responses are far more accurate and granular. But they are also far harder to analyze.
Thousands of unstructured data elements cannot be easily handled by a few quick functions in a spreadsheet. There are methods such as pattern and syntax analysis, for example, but these require more work and/or fancier analytic tools. The alternative is to go line by line and manually find patterns and put the responses into buckets such as “multiple”, “none” and “that is not a fruit”.
Strengths and weaknesses of structured and unstructured data
Structured data shines when feeding data metrics, driving automated processes, comparing options, and providing a foundation of understanding. Because the data set is limited, all the potential outcomes can be easily mapped, graphed, and counted. Structured data is also easy and fast for responders, allowing them to provide insight with very little time and effort thereby increasing the chance that they will participate.
Structured data is limited by the options provided. If the respondents answer isn’t represented in the options, it’s not counted (such as those kiwi lovers out there).
It’s also limited by the quality of the questions. Your data set will show you which of the four is a favorite, but it tells you nothing about if the respondents would spend money to purchase the fruit or if they would favor products flavored by the fruit, for example.
Unstructured data captures details, nuances, and can identify new questions or angles for consideration. It allows you to capture opinions, suggestions, exceptions, and direction.
Unstructured data is limited by the lack of patterns or extra work it takes to recognize and analyze those patterns. It also has the challenge of respondents not being bound and focused into a single area. So, if the questions aren’t clear on their purpose, or the respondents don’t want to answer those questions (such as rant on how vegetables are better than fruit so you should ask about celery and kale), you will end up with a lot of information with minimal value. Unstructured data is also more demanding for responders, demanding more time and effort and reducing the chance that they will participate.
How to use structured and unstructured data in feedback
Use structured data to provide context and focus. Questions that use scales, single- and multiple-choice questions that are fast and easy to answer.
Use unstructured data as optional follow-up questions to get details, explanations, and suggestions.
For example:
Structured question: “From a rating of 1 – 4, How helpful did you find the interview FAQ in preparing for the interview where 1 is ‘not at all helpful’ and 4 is ‘I knew exactly what to expect.’”
Unstructured optional follow-up: If the respondent answered 1-2 ask “What information can we provide in the FAQ that would make it more helpful in preparing for the interview process?”
Bringing it all together
Collecting feedback is a balance of the high-level understanding of what is going on, and detailed analysis of why or what can be done better. For this, the team of structured and unstructured data, when used correctly, can combine the strengths of fast, easily understood information with depth of value that respondents have to offer.
Comments